A heavy reliance on digital technology and systems that we don’t fully understand has eroded our digital trust. We are both concerned and confused by issues of data privacy, fake news, automated decision making and artificial intelligence. How did we get here and how can we rebuild trust?
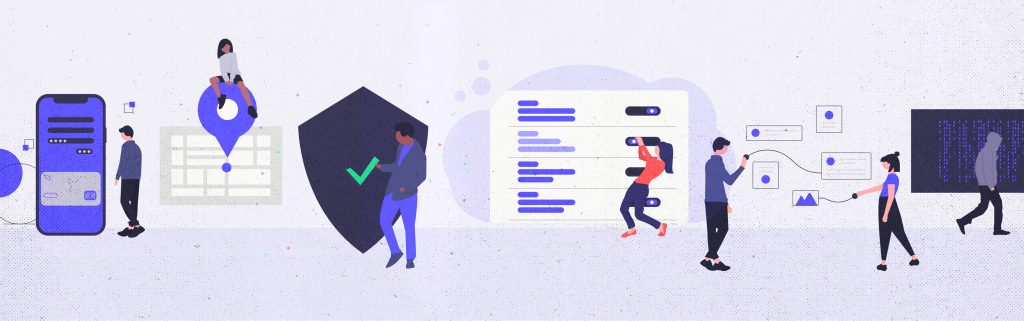
Cast your mind back to those halcyon days of the web in its infancy. Dial-up, AOL Instant Messenger, chat rooms, Napster. Sure the connections were unreliable and download speeds pitiful but things felt optimistic. There was an excitement to being able to instantly connect with millions of other people across the globe for the first time. The early internet was a wild west, there were no rules, no established conventions of engagement, just a utopian outlook on what could be.
We happily chatted anonymously with complete strangers in chat rooms, we spent hours downloading files on Napster purporting to be our favourite songs. Large corporations were slow to enter the land grab and the gold rush was led by many independent prospectors.
That utopian optimism required very little trust from users. It was enough just to experience new things online, we didn’t expect much practical use from it. Serious e-commerce and media consumption were still a long way off.
Jump 30 years forward to the present day. That utopian optimism has all but worn off. We’ve been through a dot com bubble and now internet giants are some of the most powerful companies in the world. Those companies and many others in Silicon Valley were founded upon utopian ideals — that with the right technology and the power of the web we could improve the world. We trusted these new, youthful companies. They made our lives easier and were a welcome contrast to the sluggish, old corporations who still seemed reluctant to digital change — dinosaurs who steadfastly refused to acknowledge the meteor in the sky.
Many of these internet giants were built on the foundational principle of ‘Ask for forgiveness, not permission’. In some ways they had to be. Developments online move faster than the speed of legislation. Lawmakers are constantly playing catch up. However we are now seeing the impacts of this approach writ large and the time has finally come for these companies to ask for forgiveness.
Simply put, we have trust issues.
Triggered by a series of high profile data leaks, hacks, misuses of power and unbridled political influence, we are now seeing the tide turning in our attitudes toward Silicon Valley and the tech giants. In Europe, stricter regulations have been introduced regarding the use of personal data (GDPR). The influence of social media and targeted advertising on voters is a regular topic during elections. Last year, Facebook co-founder Chris Hughes called for a break up of Facebook’s monopoly. More recently, social media platforms have come under fire for their reluctance to take responsibility for the content their users post.
This is only the beginning. We are now on the cusp of the 4th industrial revolution with automation and machine learning playing an ever more present role in our lives. In this climate, rebuilding dwindling trust with consumers is a real focus. Let’s look at four factors that have influenced this loss of trust and what businesses can do to regain it.
1. Permission
The value exchange between the internet giants and consumers has, so far, been predominantly: the access to convenient services in exchange for customers’ data — or at the very least, their attention. If a service is convenient enough, then consumers are prepared to pay, although the freemium model still looms large — with the few premium customers funding the basic usage of the many. The general rule with many online services is: “if a product or service is free…you are the product.” It should be noted that this hasn’t always been the case, with the open source approaches of Linux, Mozilla and Wikipedia as examples. Consumers have, until recently, been happy to give away seemingly worthless personal data in exchange for free services to enhance their lives. Silicon valley giants at first seemed like benevolent organisations providing us with brilliant new free services. However, generations have now matured and various misuses of data, such as the Facebook/Cambridge Analytica scandal, have revealed the potential power that this information enables. Consumers have become more conscious of giving away their data and the introduction of GDPR in Europe has started to defend the right to data privacy. What trust had been built up by the gifting of free services may just as quickly be eroded by the misuse of data and the failure to gain sufficient user permissions. Data privacy and permissions will continue to be a theme that affects our trust in the companies with which we deal daily. Recently, there has been competition amongst major players for the title of ‘people’s data champion’ — with Apple leading the way with a series of billboard ads about privacy at 2019’s CES expo in Las Vegas.
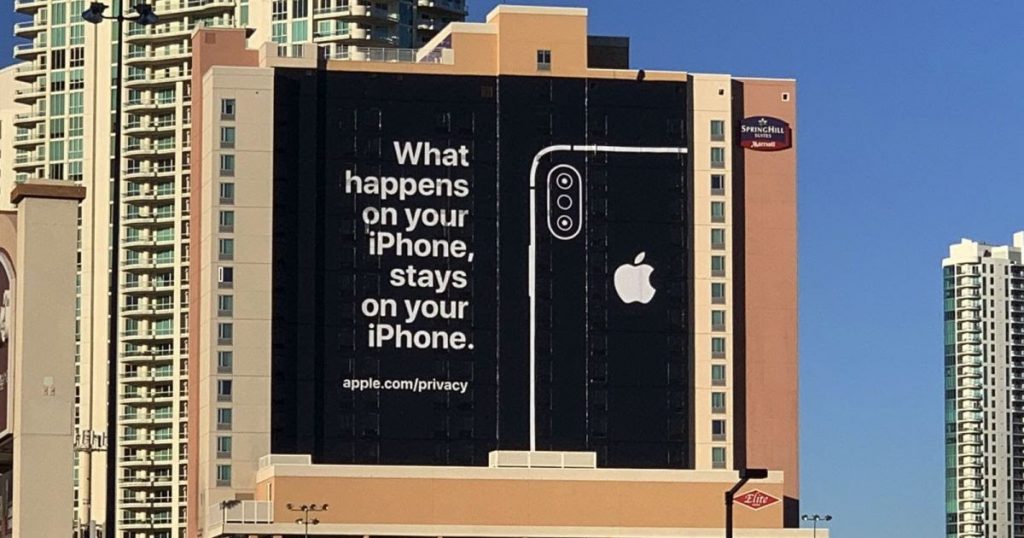
Designing to address Permissions:
- Ask for user permissions
- Be clear and transparent about what the data will be used for
- Explain if data will be accessible to third parties
- Allow users the ability to maintain control of their data permissions over time
2. The knowledge gap
Arthur C Clarke once wrote in one of his 3 Laws that “Any sufficiently advanced technology is indistinguishable from magic.” We are increasingly surrounded by magic and there’s no evidence that our technical literacy is keeping pace with the advancements in this magic. Our jobs have become more specialised and narrow in focus. The rate of change and innovation is ever-increasing, things will only become more complex. The effect of all of this change is a widening knowledge gap. Try opening up the hood of a new car and see how much of it you can tinker with. Try opening up your Apple laptop and installing new RAM. We are increasingly facing products and services with ‘no user-serviceable parts’. This therefore calls into question our trust for the products and services we use. Do we need to understand them in order to use them? If we don’t understand how they work, how can we be sure they are working in our best interests? There is a growing movement around the ‘Right to Repair’ which aims to hold manufacturers to account. The movement centres around issues of sustainability, product lifecycles and access to information. The EU has brought in rules around the right to repair for white goods and is now looking at the same for consumer electronics.
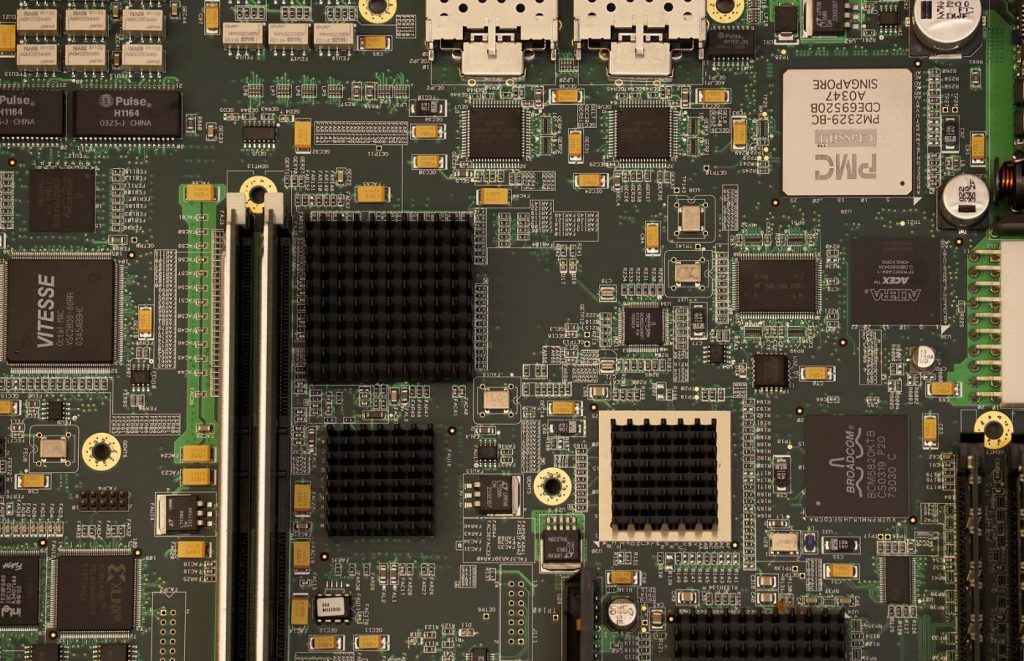
Since we are not able to bridge the knowledge gap alone, we must rely on the guidance of subject matter specialists from a wide range of sectors. This requires trust in the experts who can help us cross the knowledge gap . Member of parliament in the UK Michael Gove was famously quoted as saying: “I think the people in this country have had enough of experts with organisations from acronyms saying that they know what is best and getting it consistently wrong.”, indicating a growing scepticism of expert opinions. We are seeing the rise of vaccine hesitancy, which the World Health Organisation listed as one of its ‘Ten threats to global health in 2019’. In 2020 one of the WHO’s Urgent Health Challenges is. ‘Earning public trust’.
“Public health is compromised by the uncontrolled dissemination of misinformation in social media, as well as through an erosion of trust in public institutions. The anti-vaccination movement has been a significant factor in the rise of deaths in preventable diseases.”
– Urgent health challenges for the next decade, World Health Organisation
13 January 2020
The gatekeepers of information have a duty to surface the highest quality information. Should we trust the information that is tailored and surfaced to us based on our behaviours and interests? Does this not serve to grow the knowledge gap rather than reduce it? Surfacing information based on our preferences and behaviours could force us deeper into our filter bubbles rather than expanding our horizons and widening our knowledge base.
Designing to address The Knowledge Gap:
- Provide educational materials, strive to break down complex topics with clear explanations that are readily accessible
- Surface information from neutral sources where possible to build trust
- Reference the provenance of the information surfaced
- Provide information about the publisher, author or subject matter experts, their background and credentials
- Be transparent about any potential conflicts of interest
3. The black box effect
A knowledge gap is one thing, but at the very least we might be aware of the knowledge we lack — the ‘known unknowns’. Another area that is growing at a rapid rate is the somewhat more stealthy ‘unknown unknowns’ — issues or workings of which we’re not even aware.
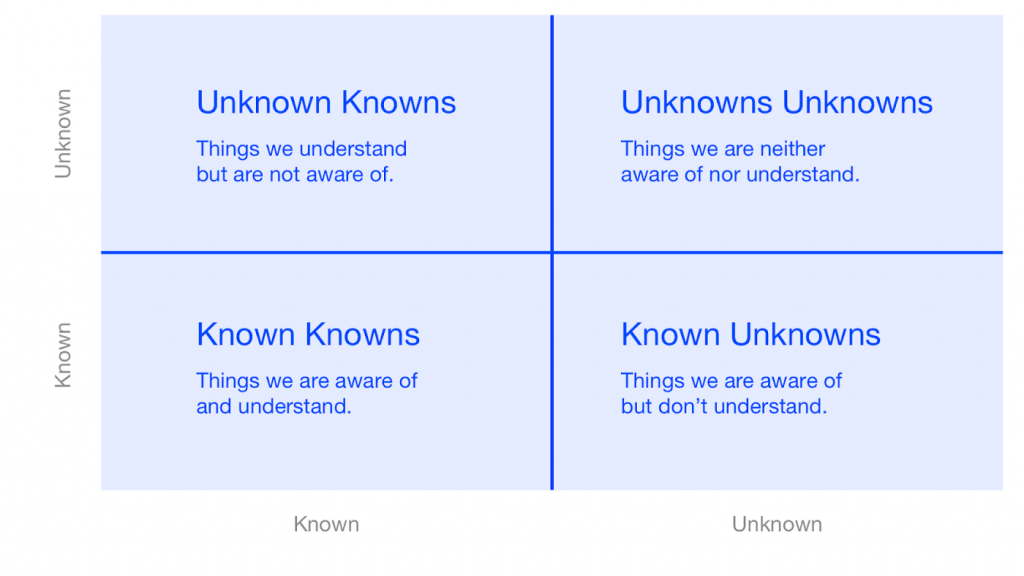
So much of our digital lives are shaped and guided by algorithms and, increasingly, machine learning. We trust the outcomes and results handed to us by digital services, often without understanding how they were generated or awareness that they have been tailored to us.
We may all have a sense that the internet is a single universal platform and our experiences online are similar. However we each live in a customised, tailored filter bubble that spreads much wider than obvious areas like our Facebook newsfeeds.
Our behaviours online trigger data points which in turn affect our tailored experiences. When you watch a show on Netflix, your future recommendations and even the thumbnail images used to promote other content are customised to meet your preferences. Thumbnail images may be selected to appeal more to users who show a preference for comedy, yet different thumbnails for the same content may be selected to appeal more to lovers of thrillers.
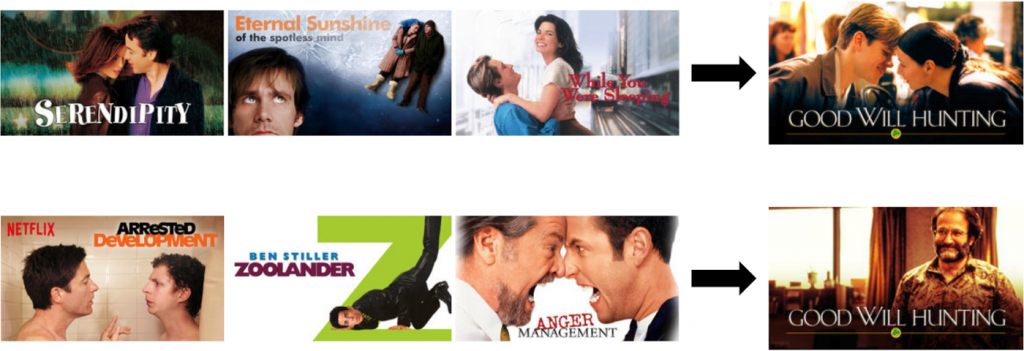
There is often a lot more going on under the hood of the services we use daily. We trust the outcomes of these systems if they work well for us, but do we really understand what drives them and what happens when something goes wrong?
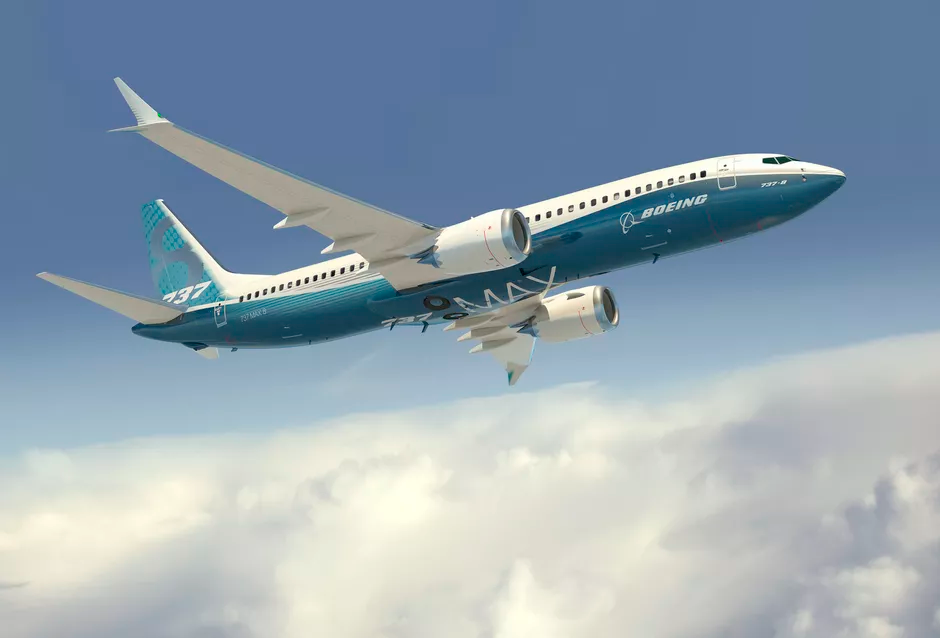
In March 2019, the Boeing 737 MAX 8 passenger airliner was grounded worldwide after 346 people died in two crashes involving the plane. Boeing later revealed that a new automated flight control, the Maneuvering Characteristics Augmentation System (MCAS), could mistakenly force the aircraft into nosedives. Boeing had omitted an explanation of MCAS from crew manuals and training. This was a situation where a software system had been introduced to fix a mechanical balancing issue with the plane, yet the pilots flying the plane had no idea it even existed, nor were they trained to deal with it. For these pilots, they were dealing with an ‘unknown unknown’.
As machine learning starts to augment the systems we create, a new set of problems relating to trust begins to emerge. The first of these problems is around unexpected outcomes. Systems that use machine learning need to be trained on existing data in order to hone the outcomes they produce. The quality or suitability of the data used to train them can affect the nature of their outputs. If we train the system on the wrong data, it may not produce the desired results. It’s at this stage where we have the potential to bake in existing biases. Biases of which we may not be consciously aware.
In 2015 Amazon cancelled an experimental hiring tool which used artificial intelligence to rate job candidates. The system had been trained on the company’s historical hiring data — teaching the system to recognise candidates that most closely matched previous successful hires. Most of these hires tended to be men, a reflection of male dominance across the tech industry, and therefore the system had unwittingly been trained to favour male resumes. It penalised resumes that included the word “women’s,” as in “women’s chess club captain.” And it downgraded graduates of two all-women’s colleges. Ultimately the tool was never used by Amazon recruiters to evaluate candidates, however the lesson still stands. In order to build trusted systems we must be conscious from their conception of any biases or negative inputs that may translate unforeseen outcomes.
Another potential problem relating to trust in AI systems is the ‘black box effect’. In the previous example, Amazon could clearly see the relationship between the biased input data and the biased outputs of their system. However, in a black box system we can see the inputs and outputs but we have no visibility of the inner workings. How can we trust the outputs when we don’t know how or why they have been generated? One could argue that, as long as the outputs meet the desired outcomes, do we really need to know the methods by which they’ve been derived? If it works often enough to be useful, then isn’t that good enough? This logic doesn’t really hold up when we’re dealing with key decision-making scenarios such as those in a healthcare or mechanical safety context. If AI will be powering our medical diagnoses or our self-driving cars — life or death situations, then we’ll need systems that we trust to a degree higher than simply ‘good enough’.
One example of this can be seen in work conducted by Google’s AI researchers using retina scans. After analysing retina scans from over a quarter of a million patients, the neural network the team developed can predict the patient’s age (within a 4-year range), gender, smoking status, blood pressure, body mass index, and risk of cardiovascular disease.
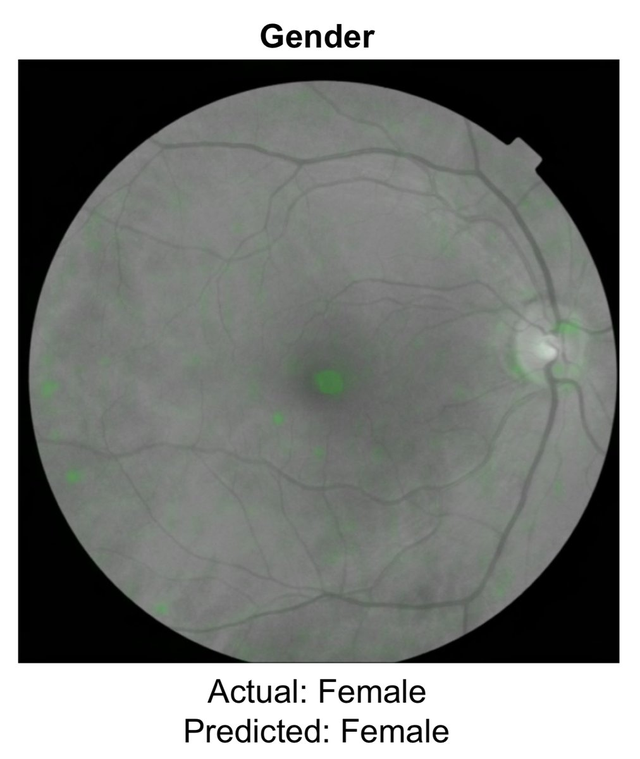
If a human doctor looked at a retinal photo, their chance of correctly identifying the patient’s gender would be 50–50, that is to say, a complete guess. There are no visual cues that the doctor could use to help them identify the gender correctly. Google’s system with its deep learning training led to a prediction accuracy of 97%. However, while the system appears accurate, there is no way of opening up the black box to understand what the system is ‘seeing’ that we as humans are unable to see ourselves. The AI is statistically highly accurate in its predictions yet we have no way of understanding its methods.
The question we should ask ourselves is, would you trust a highly accurate prediction if you had no way of knowing how it was derived? How is this different from putting your trust in a human expert in a field outside your understanding?
In Europe, GDPR already has some rules limiting how automated decision making can be used. Article 22 of the GDPR states that:
“The data subject should have the right not to be subject to a decision, which may include a measure, evaluating personal aspects relating to him or her which is based solely on automated processing and which produces legal effects concerning him or her or similarly significantly affects him or her, such as automatic refusal of an online credit application or e-recruiting practices without any human intervention.”
Some commentators maintain that being able to interrogate an AI about how it reaches its outputs should be a fundamental legal right, this is known as the “Right to Explanation”. The requirement that companies should be able to explain to users how decisions are reached by these systems becomes more difficult as the use of AI increases in complexity. This requirement may not even be possible. The computers that run services that use deep learning in their processes have likely programmed themselves in ways that humans cannot understand. Often the engineers who build these systems cannot fully explain what is actually happening inside these black boxes.
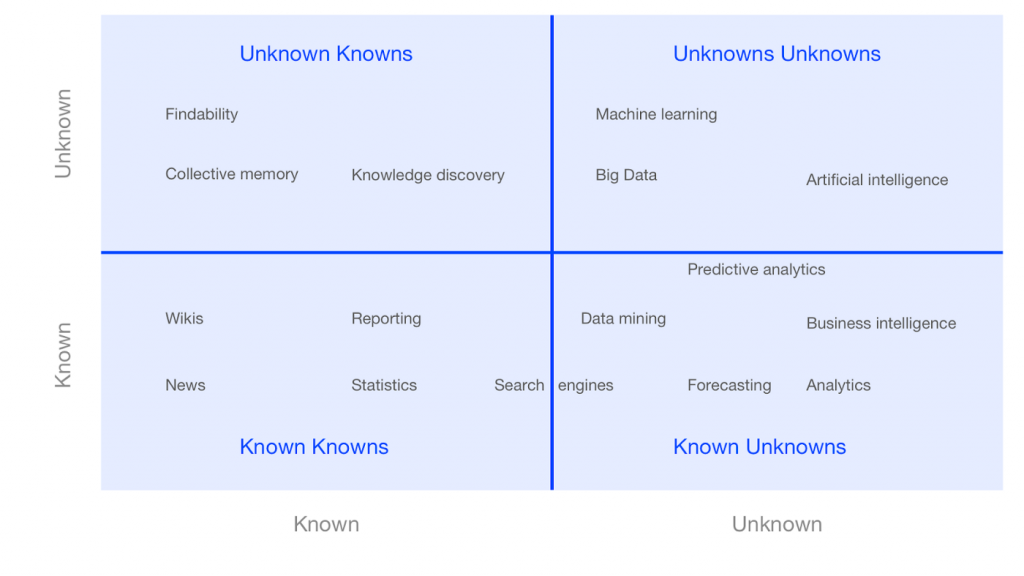
This raises some difficult questions. As technology becomes more advanced we might soon have to relinquish control and take a leap of faith. As humans, we can’t always explain our thought processes, yet we find ways to operate on a level of trust in spite of this. Ultimately we at least understand in general how human thought operates. We may need to adopt a similar approach when it comes to AI, even if these systems fundamentally process information very differently from us. For the first time we are now creating machines where we no longer control the outcomes. How we learn to trust these outcomes will define our relationships with technology going forward.
A growing reliance on technology and systems that we don’t fully understand contributes to what is now being called “Intellectual Debt”. Not understanding why something works doesn’t stop us from making use of it in the short term, however as time progresses the gaps in our knowledge compound and we may grow more dependent on these black box technologies.
Designing to address The Black Box Effect:
- Don’t rely solely on automated decision making
- Be transparent about methodologies used to create automated systems
- Be aware of biases in AI training data
- Create an open dialogue on AI ethics
4. Provenance
Our digital lives now move so fast that it’s not unusual for us to let our guard down, particularly in familiar contexts. Many of the largest online platforms we use act as an aggregator of content. We have an inherent trust in the first page of results that Google serves back to us, we trust that the PageRank algorithm is doing its job well. A product is legitimised by being available via Amazon, even if we know nothing about its manufacturer or vendor.
Amazon often recommends products from its Amazon Basics range ahead of private label products, you’ve probably come across these before. When you’re offered these products, you recognise the Amazon name and that you’re shopping on their platform and make the instant association between the vendor and their ‘store brand’ product. What you might be less aware of is the multitude of other brands offered in Amazon search results that are also owned by Amazon. The provenance of these brands is obscured, their link to Amazon is not signposted.
Silicon Valley giants are now the gatekeepers of so much of our online activity, how can we better understand the provenance of the information they surface to us? How can we better understand how their algorithms decide what we see and when?
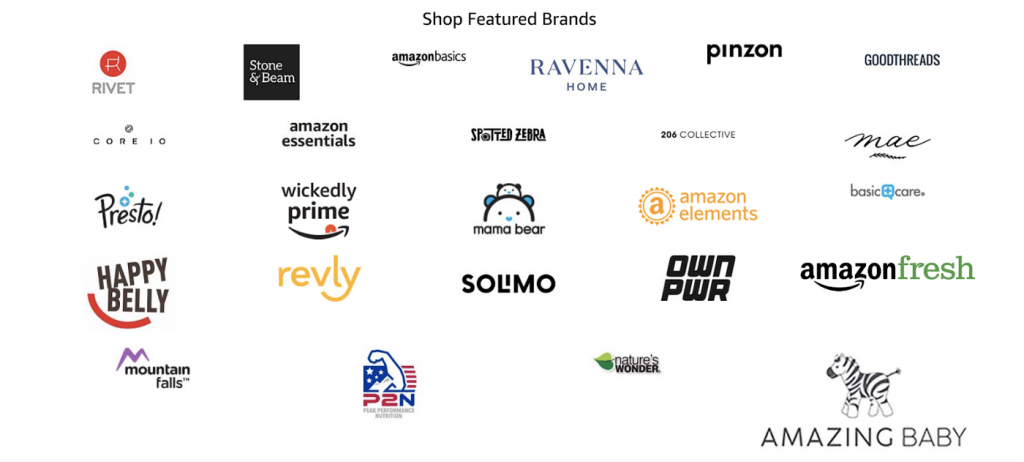
All articles linked on Facebook have the same prominence in the newsfeed, and motivation to click on an article is likely driven more by its headline and thumbnail image than the source of the content. The platform legitimises the content. We now inherently trust what these platforms serve us and this can lead to abuses of that trust.
The origin of a product or a piece of content can be a qualifier of trust, provenance matters. Fake News relies on users not checking the source or author of their content. Increasingly lines of trust are being drawn across national borders.
There is an ongoing debate around social media platforms in particular. Should these platforms be viewed as mere aggregators, with no responsibility for the content their users post, or should they be viewed as publishers, who have a responsibility for the content they host? Should Facebook be held accountable for the content their 2.6 billion users post? Is it feasible to manage that scale of content being published?
Twitter has recently made headlines for adding fact check alerts and limiting visibility on some of Donald Trump’s more controversial tweets. This has brought the platform vs content debate to the forefront once again.
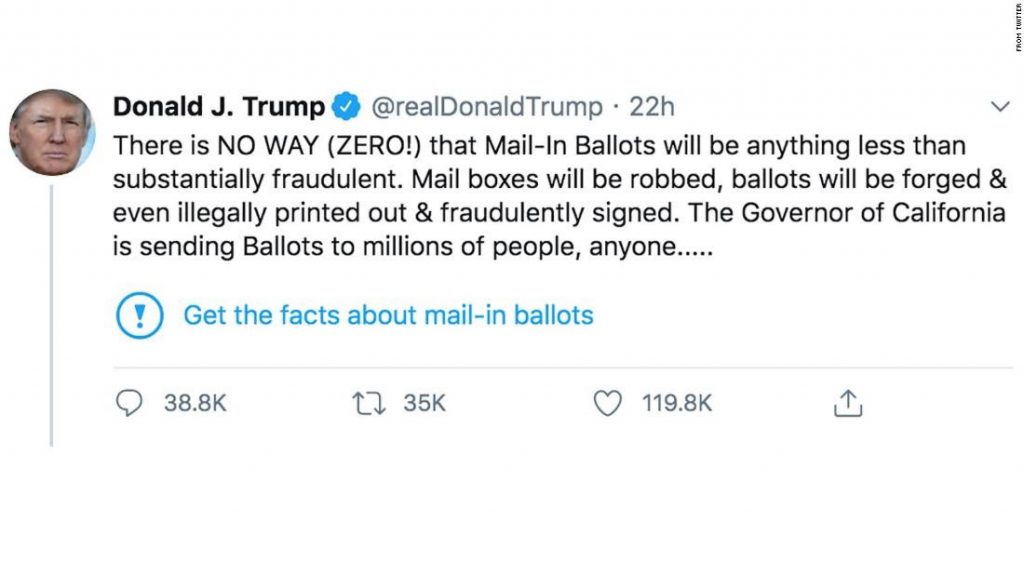
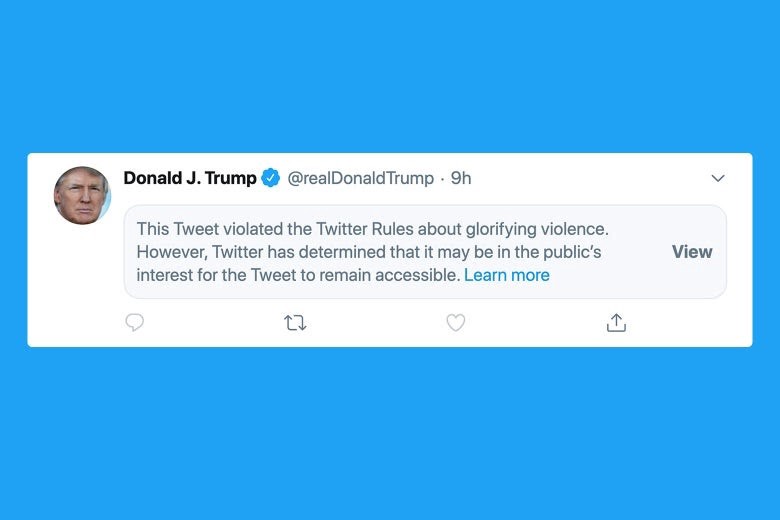
Meanwhile Facebook has refused to act similarly on the same content posted on their platform. With CEO Mark Zuckerberg stating: “I disagree strongly with how the President spoke about this, but I believe people should be able to see this for themselves, because ultimately accountability for those in positions of power can only happen when their speech is scrutinized out in the open.”
Many companies are making efforts to use provenance as a qualifier for trust. YouTube has begun to qualify sources, particularly of news content with a link to more information about the publisher of a video.
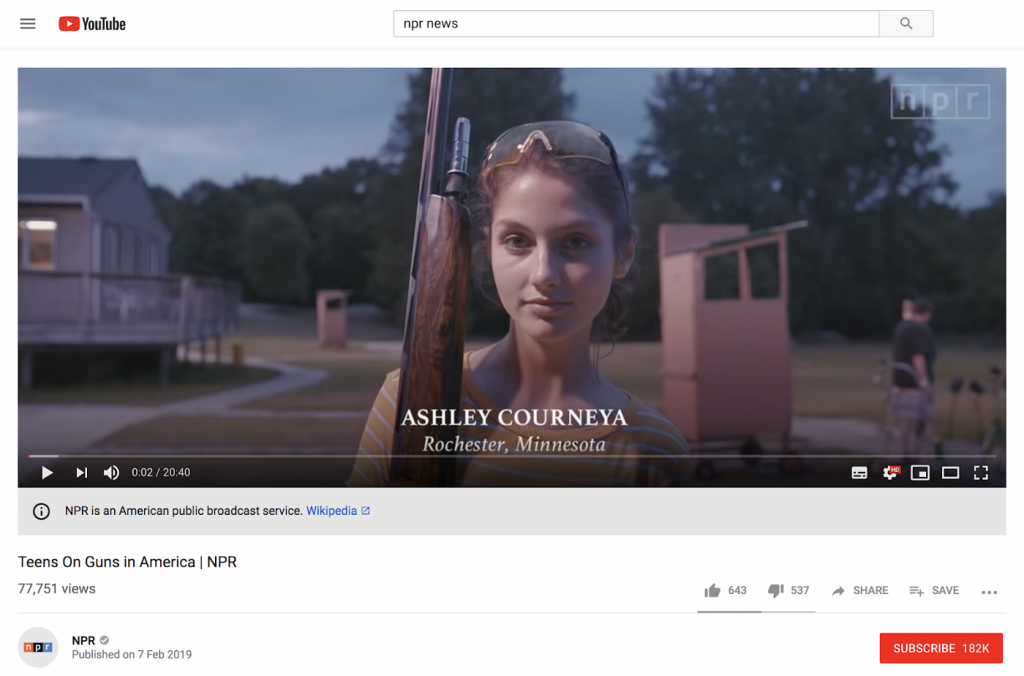
Designing to address Provenance:
- Provide contextual references and citations for information
- Provide information about publishers and authors
- Signpost why information is being surfaced
- Warn users of potential issues with the information
- Be transparent about any potential conflicts of interest
In Conclusion
While users’ trust has been eroded through the irresponsible actions of technology providers, there is now a chance to rebuild this trust through better business practices and legislation. New companies and startups have the chance to learn from the mistakes of their more established competitors, focusing on permissions, transparency and provenance from day one. Companies who have failed on these fronts in the past now need to double down on them in order to regain the trust of their users, or risk losing out to those that do. Trust is not a competitive advantage, it’s a prerequisite.